Retinal surgery is an excellent example of a high-demand dexterity procedure that may benefit from robotic systems. However, due to an incomplete understanding of a surgeon’s imminent intentional manipulations, the assistance provided to the surgeons by current robotic frameworks is a “passive” support, e.g., by damping hand tremor, which is not able to predict and/or prevent unintentional maneuvers by the surgeon. Therefore, in this paper, a novel active system, active interventional control framework (AICF), is presented to enable prediction and prevention of unintentional and potentially risky maneuvers of the surgeon, i.e., exertion of excessive forces to the sclera in retinal surgery. AICF consists of four components: 1) the steady-hand eye robot as the robotic module; 2) a sensorized tool to measure tool-to-sclera forces; 3) a recurrent neural network to predict occurrence of undesired events based on a short history of time series of sensor measurements; and 4) a variable admittance controller to command the robot away from the undesired instances. A set of user studies were conducted involving 14 participants (with 4 surgeons) to perform a vessel-following task on an eyeball phantom with the assistance of AICF as well as other two benchmark approaches, i.e., auditory feedback (AF) and real-time force feedback (RF). Statistical analysist shows that AICF results in a significant reduction of proportion of undesired instances to about 2.5%, compared to 38.4% and 26.2% using AF and RF, respectively, which proves that AICF can effectively predict excessive-force instances and augment performance of the user to avoid undesired events during robot-assisted microsurgical tasks. The proposed system may be extended to other fields of microsurgery and may potentially reduce tissue injury.
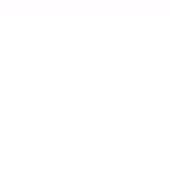