While the term “image-guided surgery” has gained popularity fairly recently, the use of imaging for medical interventions dates as far back as the beginning of the 20th century. Dr. George H. Gray of Lynn, Massachusetts, reported in his 1908 article “X-rays in Surgical Work,” published in volume 2 of the Journal of Therapeutics and Dietetics, that “the one great stride in the handling of difficult cases was the accurate diagnosis made possible by the use of the X-rays.” His story points to the day when a seamstress presented to his office with a broken sewing needle embedded in her hand. Thanks to the use of the recently discovered X-rays by Wilhelm Conrad Roentgen, the father of diagnostic radiology, Gray was able not only to confirm that the needle was indeed embedded in her hand but also to locate its parts, saving “an hour’s hunting as some had previously done and then often failed.”
Several years later, Victor A.H. Horsley and Robert H. Clarke reported on the concept later referred to as stereotaxy, which allowed the association of a coordinate system to a monkey’s head using external markers as reference points. This device, then named the “stereotactic frame,” was useful for providing a convenient reference point to establish the spatial relationship between patient and image. The concept was later adopted for human use, with stereotactic frames still employed today in some neurosurgical procedures. In many cases, however, their use has been replaced by so-called “frameless stereotaxy,” which relies on other, noninvasive approaches to perform registration and establish the transformation between the image and patient coordinate systems.
Following nearly 100 years of developments in computing, medical image acquisition, and visualization technology, surgical treatments that were traditionally conducted under highly invasive circumstances are now routinely performed using less invasive approaches. While surgery has traditionally involved the exposure of the affected organ via typically large incisions, image guidance has, for the most part, introduced less invasive alternatives for performing traditional interventions. A good example is the wide variety of thoracoabdominal procedures that relied on large incisions to access, see, and manipulate the internal organs but are now performed using laparoscopic or robotic technology. The only remnants that remind patients they once underwent a surgical procedure are small scars through which a laparoscopic camera and several surgical instruments were inserted to access the targets that required treatment.
Concurrent with technological and computational advances, two notable changes occurred in medicine during the past half century: the introduction of endoscopic imaging [1] and the establishment of interventional radiology as a surgical subspecialty [2]. The former significantly reduced the size of the incision by enabling visualization via miniature cameras inserted through small ports, while the latter showed successful percutaneous access and treatment using intraoperative imaging. Both developments represent a significant shift from traditional direct-view surgery to image-guided interventions. While this shift improves patient outcomes, the technique requires significant mental and manual dexterity on the part of clinicians to integrate the information from multiple image sources and relate this to the location of their tools with respect to the patient’s anatomy.
Current State of Technology
The goal of the current generation of image-guided intervention systems is to increase the clinician’s spatial navigation abilities while reducing the mental burden associated with fusing multiple sources of information. This is achieved using a number of key technologies including intraoperative imaging, which has continued to improve. X-rays are used to reconstruct three-dimensional (3-D) anatomical representations intraoperatively with cone-beam computed tomography (CBCT) or to guide catheters and other devices through the vasculature under continuous twodimensional fluoroscopic imaging. Similarly, ultrasound (US) imaging provides 3-D and four-dimensional (3-D plus time) imaging of dynamic structures and has become the standard of care for monitoring and guiding several complex interventions, some relying solely on this modality for guidance and visualization.
In addition, supporting technologies have also made tremendous strides over the past two decades. Optical and electromagnetic tool localization and tracking techniques have become clinically accepted, and sophisticated visualization systems have moved from being expensive research tools to becoming commodity items. Clinicians can use high-quality diagnostic computed tomography (CT) or magnetic resonance imaging (MRI) images to plan the intervention, fuse them with images acquired during the intervention (using endoscopy, real-time X-ray, or US imaging), and use global positioning system (GPS)-like technology to locate and navigate the surgical instruments in the image guidance environment.
As such, the typical image guidance and navigation workflow entails several processes, including preoperative medical imaging and modeling, intraoperative imaging, surgical instrument localization, image/model-to-patient registration, multimodality image fusion, visualization and display, navigation and therapy delivery, and, lastly, verification and validation. These processes and their interdependent relationship within the image guidance workflow are schematically represented in Figure 1.
As emphasized by the illustrated workflow, the navigation of the surgical tool to the target is as important as the task of positioning it on target, and different imaging technologies need to be employed for each phase. In addition, intuitive user interfaces have the potential to decrease the level of cognitive load experienced by the surgeon and positively impact the outcome of the procedure, catalyzing the adoption of image-guided therapy platforms by the clinical community.
A recent book by Ferenc Jolesz [3], a pioneer and long-term supporter of image-guided interventions, provides a comprehensive survey of state-of-the-art image guidance techniques in various organ systems. Many of the research projects described rely on sophisticated intraoperative imaging such as MRI, CT, and positron emission tomography (PET), with many examples pertaining to the Advanced Multimodality Image-Guided Operating (AMIGO) suite at Brigham and Women’s Hospital in Boston (Figure 2). This is a unique interventional setting providing access to multiple imaging devices, including MRI, CBCT, 3-D US, and PET/CT. Further details are available at www.ncigt.org. While such intraoperative imaging facilities represent an expensive proposition for general use, they are a gold standard against which lower footprint technologies may be evaluated.
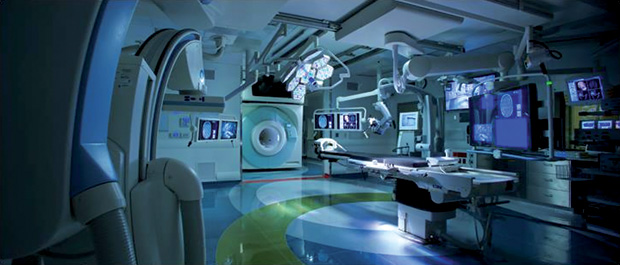
Image-Guided Therapy: What Does It Take to Make It Happen?
The development of image-guided procedures has relied on technological developments in several key areas:
- identification of the therapeutic target from medical images
- registration of images and image-derived models to the patient
- tracking of instruments with respect to the patient and inherently registered images
- assessment of discrepancies between the images and the patient
- validation of intervention accuracy intuitive information display.
Each of these tasks represents a research area in its own right that has provided countless challenges to the research community, and despite the many potential solutions disseminated in various academic venues, image-guided technology has still not experienced widespread use. The major challenges that hampered the journey from bench to bedside have pointed to limitations associated with system complexity and overall costs, with the two often intertwined.
Image-guided intervention technology is often associated with complex systems and new or modified clinical workflows. Although intended to address a current clinical hurdle, new ways of conducting routine procedures are often more challenging for clinicians. In addition, non-streamlined workflows often lead to longer procedure times with increased associated operating room costs. To ensure optimal integration into the clinic, the developed image guidance technology must enable accurate targeting of the surgical sites; render performance that does not compromise workflow, procedure duration, or interventional outcome; and provide intuitive visualization without the need for cumbersome manipulation or interaction.
Current approaches to addressing the complexity challenge can be classified into three categories. The first of these attempts to reduce information overload and streamline the clinician’s interaction with the guidance system by making the system context aware, modifying the display and interaction modes according to the needs at a particular stage of the workflow. Examples of works describing automatic stage identification in endoscopy and laparoscopy include [4] and [5].
The second category of solutions aims to develop less complex systems from the outset—a minimalistic approach. One such example is the LevelCheck system for automatic identification of vertebral levels in radiographs [6]. This system aims to help the surgeon correctly identify the targeted vertebral level, a task that can be difficult in minimally invasive surgery relying only on palpation, direct visual inspection, and the radiographic image.
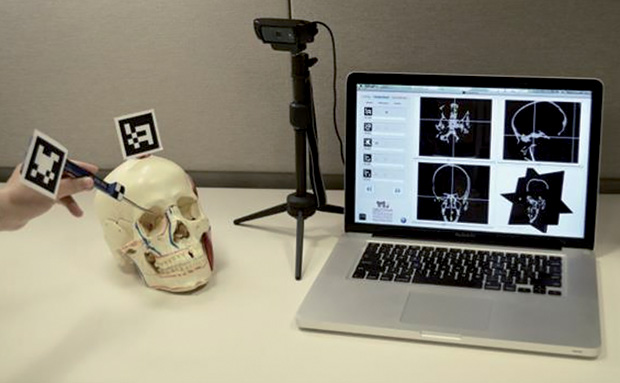
The third category entails systems aimed at providing training for image-guided workflows. For example, the Institute for Research Against Cancer of the Digestive System provides advanced training and simulation for minimally invasive surgery [7]. Nevertheless, while simulation centers address the workflow issue by providing additional training, the high costs associated with the purchase and maintenance of simulators limit their widespread use. Cost-effective simulators such as our tabletop simulator [8], shown in Figure 3, may mitigate this issue. As such, cost containment should be kept in mind from the outset, possibly by utilizing commodity hardware components when possible; an example of this is the use of red, blue, green and depth (or “RGB-D”) cameras such as the Microsoft Kinect for tracking and registration purposes, as shown in [9] and [10], in lieu of other cost-prohibitive tracking and localization solutions.
Looking Forward
Mixed-reality environments for computer-assisted intervention provide clinicians with an alternate, less invasive means for accurate and intuitive visualization by replacing or augmenting their direct view of the real world with computer graphics information (Figure 4), available thanks to the processing and manipulation of pre- and intraoperative medical images [11].
![Figure 4: A simplified diagram illustrating the use of augmented reality to display critical information [i.e., the “IGT” letters within the IGT LEGO phantom shown in (a)] to the user under conditions when such information is occluded. When the LEGO phantom is filled with rice, as shown in (b), IGT can be viewed by (c) superimposing a virtual model of the LEGO phantom generated from a CT scan onto the real video view of the physical LEGO phantom in (b) and (d) updating the image in real time according to the camera pose.](https://www.embs.org/wp-content/uploads/2016/12/linte04-2606466.jpg)
As the thrust toward greater use of minimally invasive interventions continues, image guidance will continue to be an integral component of such systems. However, wide acceptance will occur only through close partnerships between scientists and surgeons, compelling studies that conclusively demonstrate major benefits in terms of patient outcome and cost, and a commitment from the surgical device and imaging industries to support these concepts [12]. While many challenges remain, continued multidisciplinary and multi-institutional research efforts, along with ongoing improvements to imaging and computer technology, will enable a wider adoption of minimally invasive interventions while continuing to improve safety and patient quality of life.
Acknowledgment
Ziv Yaniv was supported by the Intramural Research Program of the U.S. National Institutes of Health, National Library of Medicine.
References
- G. S. Litynski, “Endoscopic surgery: The history, the pioneers,” World J. Surg., vol. 23, pp. 745–753, Aug. 1999.
- G. M. Soares and T. P. Murphy, “Clinical interventional radiology: Parallels with the evolution of general surgery,” Semin. Interv. Radiol., vol. 22, pp. 10–14, Mar. 2005.
- F. A. Jolesz, Ed., Intraoperative Imaging and Image-Guided Therapy. New York: Springer, 2014.
- O. Dergachyova, D. Bouget, A. Huaulmé, X. Morandi, and P. Jannin, “Automatic data-driven real-time segmentation and recognition of surgical workflow,” Int. J. Comput. Assist. Radiol. Surg., vol. 11, pp. 1081–1089, June 2016.
- D. Katic´, A. L. Wekerle, J. Görtler, P. Spengler, S. Bodenstedt, S. Röhl, S. Suwelack, H. G. Kenngott, M. Wagner, B. P. Müller-Stich, R. Dillmann, and S. Speidel, “Context-aware augmented reality in laparoscopic surgery,” Comput. Med. Imaging Graph., vol. 37, pp. 174–182, Mar. 2013.
- S. F. Lo, Y. Otake, V. Puvanesarajah, A. S. Wang, A. Uneri, T. De Silva, S. Vogt, G. Kleinszig, B. D. Elder, C. R. Goodwin, T. A. Kosztowski, J. A. Liauw, M. Groves, A. Bydon, D. M. Sciubba, T. F. Witham, J. P. Wolinsky, N. Aygun, Z. L. Gokaslan, and J.H. Siewerdsen, “Automatic localization of target vertebrae in spine surgery: Clinical evaluation of the LevelCheck registration algorithm,” Spine, vol. 40, pp. E476–483, Apr. 2015.
- J. Marescaux and M. Diana, “Inventing the future of surgery,” World J. Surg., vol. 39, pp. 615–622, Mar. 2015.
- Ö. Güler and Z. Yaniv, “Image-guided navigation: A cost effective practical introduction using the Image-Guided Surgery Toolkit (IGSTK),” in Proc. IEEE Annu. Int. Conf. Engineering Medicine Biology Society, 2012, pp. 6056–6059.
- P. J. Noonan, J. Howard, W. A. Hallett, and R. N. Gunn, “Repurposing the Microsoft Kinect for Windows v2 for external head motion tracking for brain PET,” Phys. Med. Biol., vol. 60, pp. 8753– 8766, Nov. 2015.
- A. Seitel, N. Bellemann, M. Hafezi, A. M. Franz, M. Servatius, A. Saffari, T. Kilgus, H.-P. Schlemmer, A. Mehrabi, B. A. Radeleff, and L. Maier-Hein, “Towards markerless navigation for percutaneous needle insertions,” Int. J. Comput. Assist. Radiol. Surg., vol. 11, pp. 107–117, Jan. 2016.
- M. Potter, A. Bensch, A. Dawson-Elli, and C. A. Linte, “Augmenting real-time video with virtual models for enhanced visualization for simulation, teaching, training and guidance,” Proc. SPIE, vol. 9416, pp. 941615, Mar. 2015.
- T. M. Peters and C. A. Linte, “Image-guided interventions and computer-integrated therapy: Quo vadis?” Med. Image Anal., vol. 33, pp. 56–63, Oct. 2016.