Shortages of psychiatrists and therapists worldwide may lead to a rise in AI solutions for mental health
An estimated 792 million people live with mental health disorders worldwide—more than one in ten people—and this number is expected to grow in the shadow of the Coronavirus disease 2019 (COVID-19) pandemic. Unfortunately, there aren’t enough mental health professionals to treat all these people. Can artificial intelligence (AI) help? While many psychiatrists have different views on this question, recent developments suggest AI may change the practice of psychiatry for both clinicians and patients.
“There are huge, huge unmet needs in psychiatry,” says Murali Doraiswamy, MD, professor of psychiatry and behavioral sciences and director of the neurocognitive disorders program at Duke University (Figure 1). “There’s an acute shortage of psychiatrists and therapists in virtually every county in the U.S. and the shortage is even more dramatic in poorer countries.”
Doraiswamy wonders whether AI technology can help fill this gap. AI algorithms can extract patterns from data, make predictions from these patterns, and continuously update the predictions with the input of new data. This means there is a wide range of potential uses for AI in psychiatry, possibly even including the diagnosis and treatment of people with mental disorders. But what do psychiatrists think about the future role of AI technology in their field?
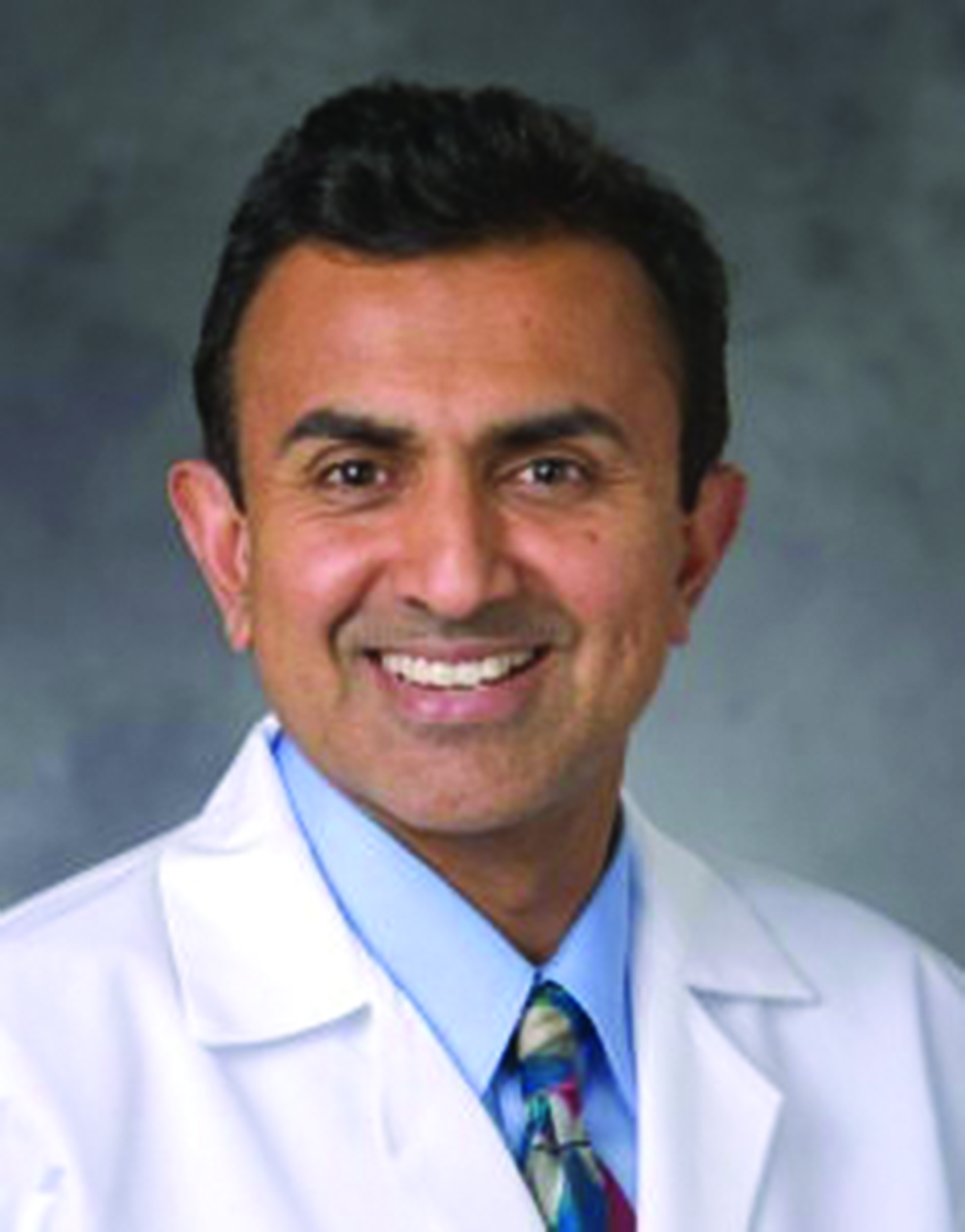
“Even the best technology will fail if the end users are not open to it,” says Doraiswamy. Doraiswamy and his colleagues Charlotte Blease and Kaylee Bodner conducted a survey of 791 psychiatrists from 22 countries using a social networking platform for physicians called Sermo [1]. The survey asked psychiatrists to predict the impact that AI would have on the field over the next 25 years.
“We asked the most provocative question, which is: do you think AI-embedded technology can replace a psychiatrist?” says Doraiswamy. “That is sort of the holy grail of AI according to many technologists.”
While nearly half of the responders predicted that AI would have a moderate influence—substantially changing—the nature of their job, only 4% predicted AI would make their jobs obsolete. The survey also asked the psychiatrists to predict the likelihood that AI tools would be able to replace the average psychiatrist in performing ten common psychiatric tasks, ranging from providing documentation (e.g., updating medical records) to providing empathetic care to synthesizing patient information to reach diagnoses.
“Really the only task that doctors were somewhat optimistic that AI could replace them was in the mundane administrative documentation types of tasks,” says Doraiswamy. While 75% of respondents thought AI tools would be able to replace updating medical records, 33% thought AI could one day perform a mental status exam, and only 17 predicted AI would be able to provide empathetic care to patients.
According to Doraiswamy, there are different ways to interpret these results. One is that doctors are correct to be skeptical about AI making their jobs—or elements of their jobs—obsolete, especially given the ways AI has been overhyped in the past 50 years. “Maybe we should not be focusing on putting our efforts into developing technology that replaces a doctor but instead focus on technology that can really enable them to work more efficiently,” he says.
Another interpretation: “maybe doctors are being defensive and maybe this is their hope, that AI won’t replace them,” he says. If that’s the case, he thinks doctors need more education to prepare themselves so that they can fully take advantage of all of the benefits of AI while also recognizing its flaws. “I think there’s a little bit of truth in both,” he concludes.
Doraiswamy notes that the psychiatrists who participated in this survey seemed eager to share their thoughts. “Virtually every doctor we gave it to responded right away; it almost seemed like they were waiting for someone to ask them these questions,” he said. “And they wrote copious notes.”
The current state of AI in psychiatry
So what are the current and potential uses for AI in psychiatry?
“In mental health care right now, I think there aren’t that many clinical frontline direct applications in use,” says John Torous, MD, director of the Division of Digital Psychiatry at Beth Israel Deaconess Medical School. “There’s a lot of interesting small efforts; none of them have become national standards or things that people always use.”
He notes that some of the projects in the works include building AI models that can predict when someone may be safe to leave the hospital or when there’s a lower risk of suicide, what medications someone could respond to, and the need for psychiatric beds.
But when it comes to aiding in diagnoses or managing patient care, AI tools may be trickier for the field of psychiatry than some other specialties. “We know that psychiatry is not like radiology or pathology, where you can do a pattern-based diagnosis,” says Doraiswamy.
For decades there’s been hope that finding biomarkers of mental disorders could make diagnosing and treating these disorders easier. Thirty years ago, the Human Genome Project offered hope; instead it revealed that there’s no single gene for depression or bipolar disorder. Next, the advent of new neuroimaging techniques garnered great excitement, but that excitement hasn’t translated into clinically useful biomarkers yet either.
“When neuroimaging first came out we were hoping that by combining the power of genomics with this new technology, we would be able to identify specific brain activity patterns that would tell us whether this person is depressed, whether this person is manic,” says Alex Leow, MD, Ph.D., associate professor of psychiatry and bioengineering at the University of Illinois College of Medicine.
More than 200 studies have used machine learning algorithms to distinguish brain differences between people with and without particular disorders such as Alzheimer’s disease, schizophrenia, and depression [2]. However, these findings haven’t been able to make the leap to clinical care.
“Somehow those patterns don’t necessarily translate to clinical use when all you care about is this specific person in front of you that you are trying to treat,” says Leow. “We are now reaching a point where we are starting to see the limitations of computational neuroimaging.”
One limitation is that neuroimaging creates a snapshot of a person’s brain activity at a particular moment in time. “I notice with myself, the way my brain functions in the morning when I wake up is very different than after I have a cup of coffee, it’s also very different than at night before I go to sleep,” says Leow. “This amount of variance is something traditional technology cannot really capture.”
Neuroimaging also requires expensive equipment and is time-consuming. “There’ve been some research studies that show a high potential for neuroimaging, but we really need things that can be scalable; we have millions of people we are trying to help, and there’ll probably be more with this pandemic, unfortunately,” says Torous. “How are we going to make sure everyone can get a diagnosis or everyone can get the right diagnostics to learn how they’re doing?”
The future of psychiatry may be in your pocket
The answer to Torous’ question might just be sitting in your pocket—that is, if you’re one of the 3.5 billion people who own a smartphone. With data from smartphone sensors, psychiatrists could have more information about how their patients are doing than they currently get from seeing patients for 30 min every three to six months.
“Can we get a deeper understanding of what the person’s true baseline is? What are the daily fluctuations? How does a person react to various situations in their life? Can we create a custom template for what depression is like for that individual?” asks Doraiswamy. “I think AI has the potential to create this whole new science of human behavior.”
Both Torous and Leow are working on projects that use smartphones to give clinicians and patients access to new information—and constantly updated information—about patients’ mental health, using a new technique called digital phenotyping [3].
Torous’ group has an open source project called mindLAMP (“Learn, Assess, Manage, Prevent”) that uses smartphones—and their embedded sensors—to help predict and understand people’s lived experience of mental illness and recovery (Figure 2) [4]. The team built their own platform to ensure data security and have been using the app in the clinic.
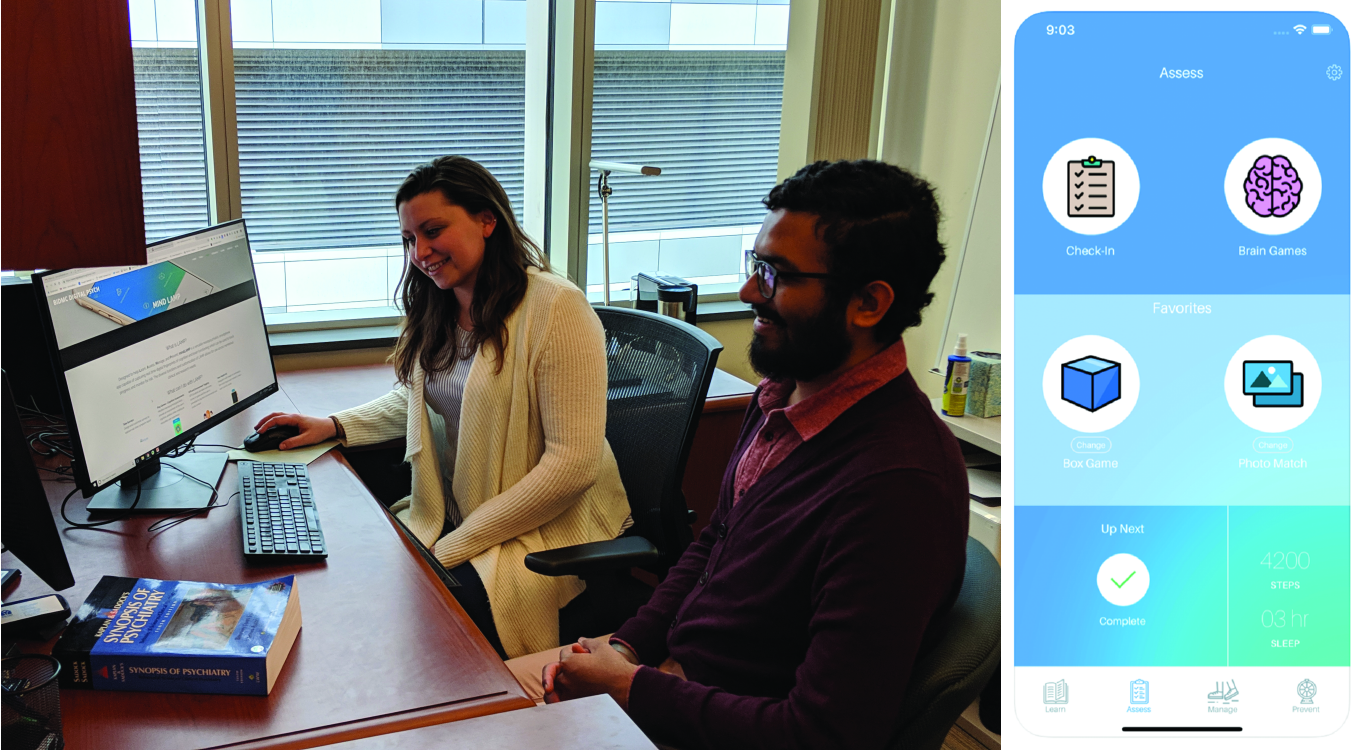
The mindLAMP app is customizable and has the ability to collect various data streams from patients such as surveys, cognitive tests, Global Positioning System (GPS) coordinates, and exercise information. A particular psychiatrist and patient can decide together which data to collect. For a person who’s started a new medication, for example, the mindLAMP app could help the patient and psychiatrist track possible side effects and mood.
Torous and his group are studying whether mindLAMP can predict relapse of serious mental illness. The idea is that machine learning algorithms may one day allow the mindLAMP app to assess the risk of relapse, notify patients and their clinical team, and recommend customized interventions in real time [5].
Already the group has used mindLAMP to see how people’s mobility patterns correlate with mental health symptoms. “We’ve shown that people who have stable mobility rhythms in their life and are adhering to those patterns, generally have lower mental health symptoms than people who have very erratic patterns,” he says.
Currently 15 institutions across the world are using the open source app as a clinical research tool. Torous says patients like having additional insight into their treatment and how they’re doing, and that the app helps build emotional self-awareness.
“You’re developing insight into how these behaviors can impact your thoughts and can impact your mental health,” says Torous.
Digital phenotyping and the democratization of patient data
Leow’s project, an app called BiAffect, takes advantage of a vital part of our smartphones that we use everyday but don’t give much thought to: the keyboard (Figure 3).
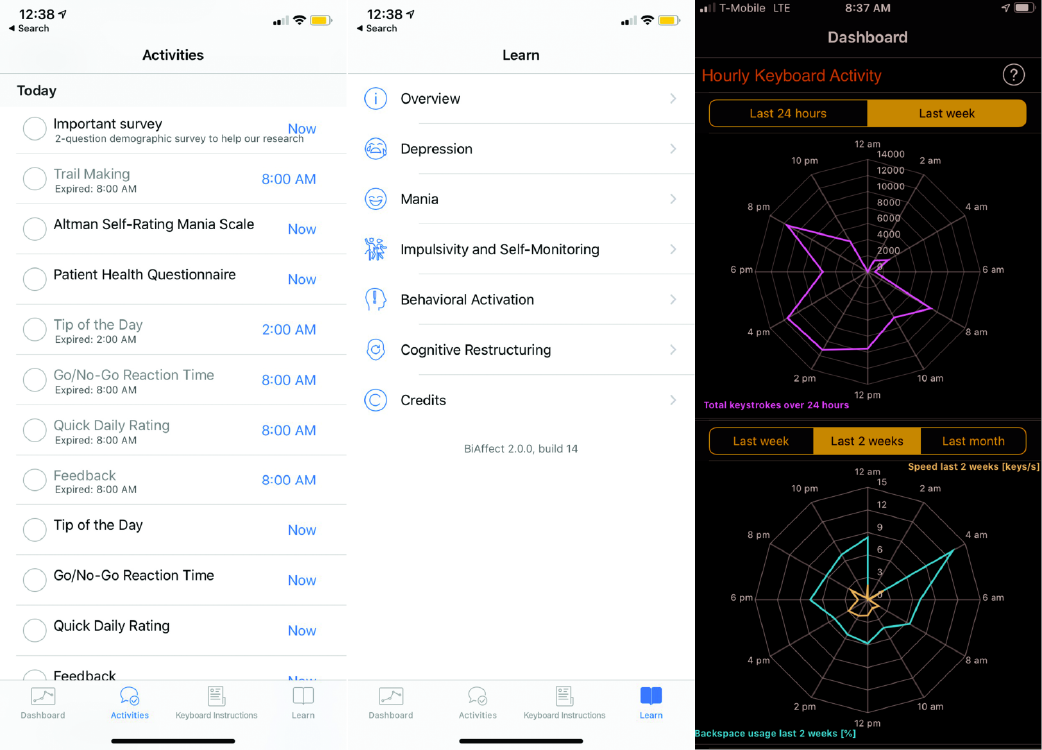
BiAffect uses machine learning algorithms to predict manic and depressive episodes in people with bipolar disorder from their keyboard metadata—variability in typing dynamics, mistakes, pauses, using the backspace key—without collecting what is typed.
Leow and her group conducted a small pilot study of people with bipolar disorder and found that mood disturbances correlated with specific changes in keyboard metadata [6]. For instance, they found that people typed faster during manic episodes—just like they talk faster—and typed shorter messages during depressive episodes.
The group has since completed a larger study, where they analyzed 15 million key presses, and are actively recruiting people with and without bipolar disorder to participate in a citizen science study via downloading the BiAffect app to better understand the relationship between mood and neurocognitive functioning.
Both mindLAMP and BiAffect show that AI has the potential to revolutionize psychiatry by making more data available to clinicians and to make the field more democratic by giving patients access to—and insights about—these data.
“Another reason why we strongly believe in taking this direction is that by leveraging sensor information, people can take that information and can formulate insights into how their brain would function differently when they do these things versus those things,” says Leow.
One day, digital phenotyping could be part of a larger systems biology approach to diagnosing mental illness. “You can use, for example, a multiclustering algorithm if you have 45 different types of information on a person—it could be genomic, could be biomarkers, could be brain scans, could be metabolomics, the microbiome,” says Doraiswamy. “No individual human can actually go through all this to try to identify patterns, it would be too complicated.”
This sort of individualized characterization could change the very nature of the field of psychiatry and the diagnosis of mental illness. “AI will enable precision psychiatry for the first time,” says Doraiswamy.
AI tools may also transform the treatment of mental disorders via the discovery of new medications and assisting in therapy [7], [8].
Toward a future of thriving
Ultimately, AI might one day be used for more than helping people manage their mental disorder symptoms—it could help prevent mental illness and even help people flourish.
“We have a really interesting target for machine learning or AI algorithms: to help us figure out what can optimize our mental health and even move towards thriving,” Torous says. “It’s a very exciting time in the field.”
References
- P. M. Doraiswamy, C. Blease, and K. Bodner, “Artificial intelligence and the future of psychiatry: Insights from a global physician survey,” Artif. Intell. Med., vol. 102, p. 101753, Jan. 2020, doi: 10.1016/j.artmed.2019.101753.
- M. R. Arbabshirani, S. Plis, J. Sui, and V. D. Calhoun, “Single subject prediction of brain disorders in neuroimaging: Promises and pitfalls,” NeuroImage, vol. 145, pp. 137–165, Jan. 2017, doi: 10.1016/
j.neuroimage.2016.02.079. - K. Huckvale, S. Venkatesh, and H. Christensen, “Toward clinical digital phenotyping: A timely opportunity to consider purpose, quality, and safety,” npj Digital Med., vol. 2, no. 1, Jun. 2019, doi: 10.1038/s41746-019-0166-1.
- J. Torous et al., “Creating a digital health smartphone app and digital phenotyping platform for mental health and diverse healthcare needs: An interdisciplinary and collaborative approach,” J. Technol. Behav. Sci., vol. 4, no. 2, pp. 73–85, Apr. 2019, doi: 10.1007/s41347-019-00095-w.
- A. Vaidyam, J. Halamka, and J. Torous, “Actionable digital phenotyping: A framework for the delivery of just-in-time and longitudinal interventions in clinical healthcare,” mHealth, vol. 5, pp. 25–25, Aug. 2019, doi: 10.21037/mhealth.2019.07.04.
- J. Zulueta et al., “Predicting mood disturbance severity with mobile phone keystroke metadata: A BiAffect digital phenotyping study,” J. Med. Internet Res., vol. 20, no. 7, Jul. 2018, doi: 10.2196/jmir.9775.
[7] G. Hessler and K.-H. Baringhaus, “Artificial intelligence in drug design,” Molecules, vol. 23, no. 10, p. 2520, Feb. 2018, doi: 10.1177/0959354319853045. - R. Fulmer, “Artificial intelligence and counseling: Four levels of implementation,” Theory Psychol., vol. 29, no. 6, pp. 807–819, Nov. 2019,
doi: 10.1177/0959354319853045.